Building a Cost-Effective LLM Pipeline: Strategies for Maximizing ROI
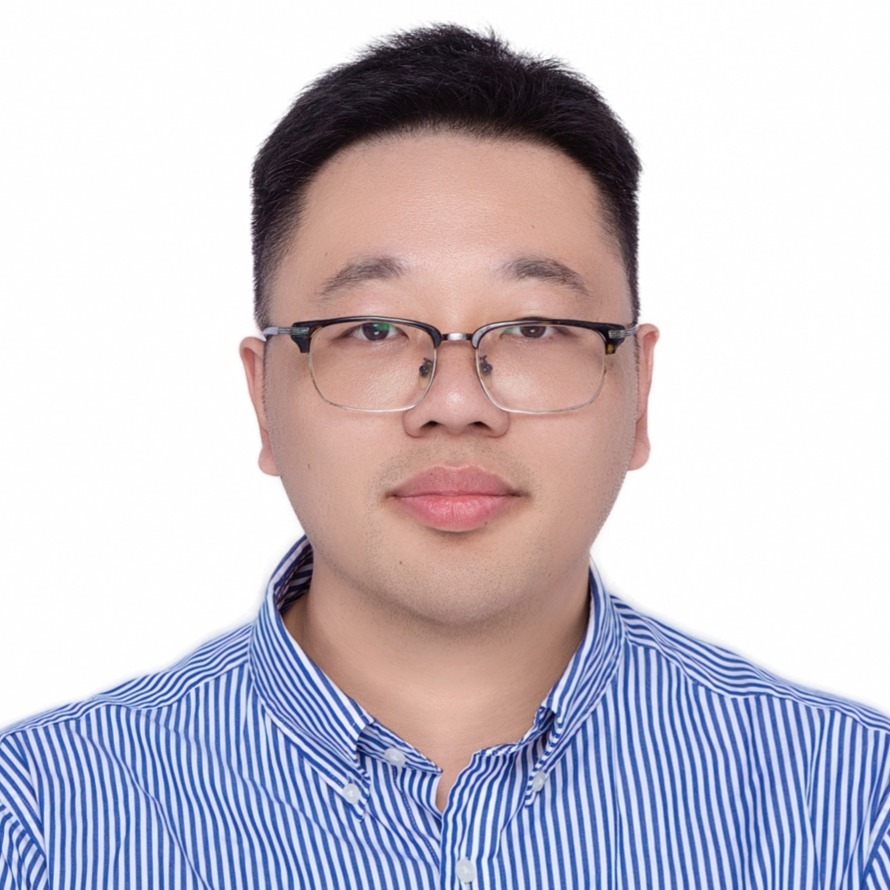
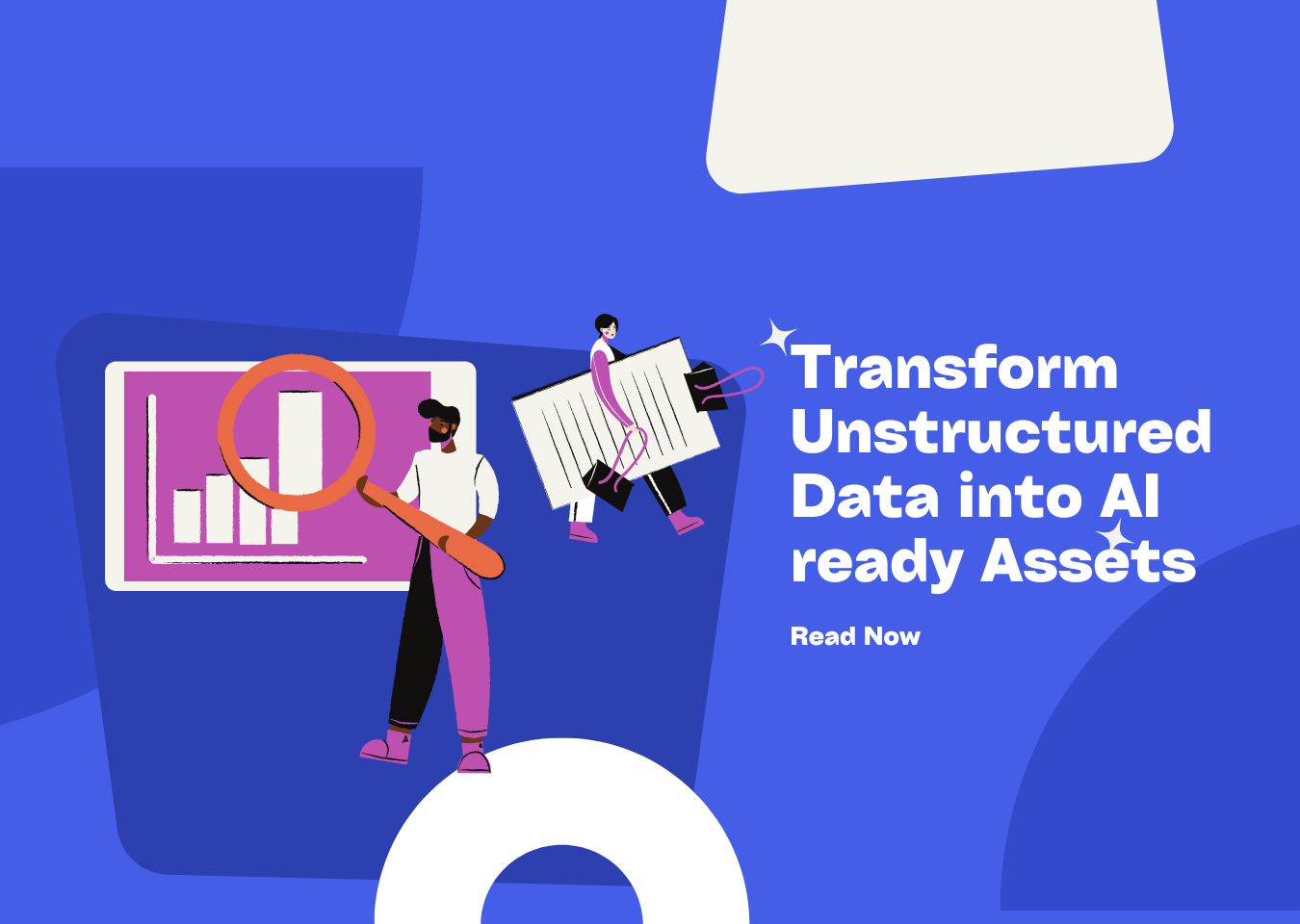
Large Language Models (LLMs) are revolutionizing industries, enabling groundbreaking applications from AI-powered chatbots and creative content generation to sophisticated code completion and in-depth data analysis. However, the extensive computational resources required for training, deploying, and maintaining these models pose a significant financial challenge. This blog post explores practical strategies for building a cost-effective LLM pipeline, facilitating broader adoption and maximizing return on investment (ROI). We’ll examine the key cost drivers, optimize model selection and inference techniques, and leverage effective tools for efficient resource management.
Section 1: Understanding the Cost Drivers in LLM Pipelines
Before embarking on optimization, it’s essential to understand the origin of costs within LLM pipelines. These pipelines comprise several stages, each contributing to the overall expense: training, inference, deployment, and maintenance.
- Training Costs: Often the most substantial expense, especially for custom models. Compute resources (GPUs, TPUs) are paramount, with costs directly proportional to training time and hardware specifications. Data storage and preprocessing also demand significant capacity and processing power. Iterative model development, including hyperparameter tuning, further increases computational demands.
- Inference Costs: This refers to the cost of generating predictions or responses using a trained LLM. Like training, inference requires GPUs or specialized hardware for real-time performance. Low-latency applications demand more resources, and scaling to handle increasing user traffic elevates costs. Larger, more complex models generally require greater computational power for inference.
- Deployment and Infrastructure Costs: The expenses associated with hosting and managing the LLM pipeline. Choosing between cloud (AWS, Azure, GCP) and on-premise infrastructure involves trade-offs between cost, scalability, and control. Monitoring, logging, and maintenance are vital for reliability but add to operational expenses. MLOps tools can streamline development but also incur costs.
Section 2: Strategies for Cost Optimization
Let’s now explore actionable strategies to reduce costs across the LLM pipeline, remembering that optimization is about balance. It’s not just about cutting costs, but about maintaining or improving performance while doing so.
-
Model Selection: Choosing the right model is critical for cost-effectiveness.
- Right-Sizing the Model for the Task: Avoid using overly large models for simple tasks. Smaller, specialized models can often deliver comparable performance at a fraction of the cost. For instance, using a massive model like GPT-4 for simple text classification is overkill; a smaller model like DistilBERT might suffice.
- Exploring Open-Source and Fine-Tuned Models: Leverage the growing ecosystem of open-source LLMs (e.g., those available on Hugging Face) and fine-tune them for your specific use case. This reduces the need to train a model from scratch. DeepSeek is a good example of a cost-effective open-source model.
- Model Compression Techniques: Employ techniques like quantization (reducing the precision of model weights), pruning (removing unimportant connections), and distillation (training a smaller “student” model to mimic a larger “teacher” model) to reduce model size and inference cost. Quantization, for example, can reduce the memory footprint of a model by 4x with minimal impact on accuracy.
-
Data Preprocessing with UndatasIO: Preparing data for LLMs can be time-consuming and expensive, especially when dealing with unstructured formats. UndatasIO streamlines this process by transforming unstructured data into AI-ready assets. Its robust parsing engine excels where other tools like unstructured.io and LlamaIndex parsers fall short, offering superior accuracy and efficiency. This is particularly beneficial for Retrieval-Augmented Generation (RAG) pipelines, ensuring your LLMs receive high-quality, context-rich information.
- Transform Unstructured Data: Convert PDFs, documents, and other unstructured formats into structured data suitable for AI applications.
- Enhance Data Quality: Improve the accuracy and reliability of your data for better LLM performance.
- Simplify RAG Pipelines: Integrate seamlessly with RAG workflows for enhanced context and reduced hallucination.
-
Prompt Engineering: Optimizing prompts can significantly impact token usage and costs.
- Crafting Efficient Prompts to Reduce Token Usage: Minimize unnecessary words and use concise language.
- Prompt Optimization Techniques: Explore techniques like few-shot learning (providing a few examples in the prompt) and chain-of-thought prompting (guiding the model to reason step-by-step) to improve accuracy and reduce the need for lengthy prompts.
- Prompt Caching Strategies: Cache frequently used prompts and their corresponding outputs to avoid redundant computations. A simple dictionary can be used to store prompts and their outputs, checking for a hit before sending the prompt to the LLM.
-
Inference Optimization: Improving inference efficiency can lead to substantial cost savings.
- Batching Requests to Improve Throughput: Process multiple requests in a single batch to amortize the overhead of model loading and inference.
- Model Serving Frameworks: Utilize optimized model serving frameworks like vLLM and NVIDIA Triton Inference Server to maximize throughput and minimize latency.
- Hardware Acceleration: Leverage GPUs or specialized AI accelerators (e.g., AWS Inferentia) to accelerate inference.
- Dynamic Scaling and Autoscaling: Automatically adjust the number of serving instances based on traffic demand to optimize resource utilization. This can be achieved using Kubernetes and tools like the Kubernetes Horizontal Pod Autoscaler (HPA).
-
Infrastructure Optimization: Optimizing your infrastructure can significantly reduce costs.
- Leveraging Serverless Computing: Utilize serverless platforms like AWS Lambda, Azure Functions, or Google Cloud Functions for event-driven LLM applications, paying only for actual usage.
- Using Spot Instances or Preemptible VMs: Utilize spot instances (AWS) or preemptible VMs (Google Cloud) for non-critical workloads to take advantage of discounted pricing. Be prepared for potential interruptions.
- Choosing the Right Cloud Region for Cost Efficiency: Select cloud regions with lower pricing for compute and storage resources.
- Data Storage Optimization: Employ cost-effective storage solutions like object storage (e.g., AWS S3) for storing large datasets.
-
Fine-tuning and Transfer Learning: Reusing pre-trained models can save significant time and resources.
- Fine-tuning Pre-trained Models on Specific Tasks: Adapt pre-trained models to your specific task with a smaller dataset, significantly reducing training costs compared to training from scratch.
- Transfer Learning from Related Domains: Transfer knowledge from related domains to improve performance and reduce the amount of data required for fine-tuning.
- Cost-Benefit Analysis of Fine-tuning vs. Using a General-Purpose Model: Carefully evaluate the trade-offs between the cost of fine-tuning and the performance gains compared to using a general-purpose model. Sometimes, fine-tuning might not yield sufficient improvement to justify the cost.
Section 3: Tools and Technologies for Cost-Effective LLM Pipelines
Several tools and technologies can aid in building and managing cost-effective LLM pipelines. These tools offer various features, from managing the LLM lifecycle to providing cost monitoring and specialized infrastructure.
- MLOps Platforms: Tools for managing the entire LLM lifecycle (training, deployment, monitoring). Examples: ZenML, MLflow, Kubeflow. These platforms provide features for experiment tracking, model versioning, and automated deployment, streamlining the development process and reducing operational overhead.
- Cost Monitoring and Analysis Tools: Tools for tracking LLM pipeline costs and identifying areas for optimization. Examples: AWS Cost Explorer, Azure Cost Management, Google Cloud Cost Management. These tools provide detailed cost breakdowns, allowing you to identify cost drivers and optimize resource allocation.
- Specialized LLM Infrastructure Providers: Companies offering optimized infrastructure for LLM training and inference. This is a rapidly growing market, with new providers emerging regularly, offering competitive pricing and specialized hardware.
Section 4: Case Studies and Examples
Real-world examples demonstrate the effectiveness of cost optimization strategies in LLM pipelines. These case studies provide tangible evidence of potential savings.
- Amazon Search: Reduced LLM training costs by 30% using AWS Trainium. This highlights the benefits of using specialized hardware accelerators.
- FrugalGPT: Achieved up to 98% cost reduction by intelligently routing requests to different LLMs based on cost and performance. This showcases the power of dynamic model selection and routing.
Section 5: The Future of Cost Optimization in LLMs
The field of cost optimization in LLMs is constantly evolving. We can expect further advancements in hardware, software, and algorithms that will drive down costs and improve efficiency. But the human element will remain important, knowing how to put these technologies to their best use.
- Further Reduction in Inference Costs (LLMflation): Continued advancements in hardware and software will drive down inference costs.
- Development of More Efficient LLM Architectures: Research into more efficient architectures will lead to models that require less computational power.
- Automated Cost Optimization Tools: AI-powered tools will automate the process of cost optimization, continuously analyzing and adjusting pipeline configurations to minimize expenses.
Conclusion
Building a cost-effective LLM pipeline is essential for realizing the full potential of these powerful models. By understanding the cost drivers, implementing the strategies outlined in this blog post, and leveraging the right tools, organizations can significantly reduce expenses and maximize their return on investment. The key is to remember that the most performant LLM isn’t always the most cost-effective; and the cheapest isn’t always the most performant, it’s about finding the sweet spot. Continuous monitoring and optimization are crucial for maintaining cost efficiency as LLM technology evolves.
Call to Action
Ready to unlock the full potential of your LLM pipeline? Visit UndatasIO today to discover how our data transformation solutions can significantly reduce your AI development costs and improve the accuracy of your LLM applications. UndatasIO website
Start implementing these strategies today to optimize your LLM pipeline costs. Explore the resources listed below to learn more and connect with the community to share your experiences and insights.
📖See Also
- In-depth Review of Mistral OCR A PDF Parsing Powerhouse Tailored for the AI Era
- Assessment-Unveiled-The-True-Capabilities-of-Fireworks-AI
- Evaluation-of-Chunkrai-Platform-Unraveling-Its-Capabilities-and-Limitations
- IBM-Docling-s-Upgrade-A-Fresh-Assessment-of-Intelligent-Document-Processing-Capabilities
- Is-SmolDocling-256M-an-OCR-Miracle-or-Just-a-Pretty-Face-An-In-depth-Review-Reveals-All
- Can-Undatasio-Really-Deliver-Superior-PDF-Parsing-Quality-Sample-Based-Evidence-Speaks
Subscribe to Our Newsletter
Get the latest updates and exclusive content delivered straight to your inbox