Taming the Chaos: A Guide to AI-Powered Data Quality Tools for Unstructured Data Transformation
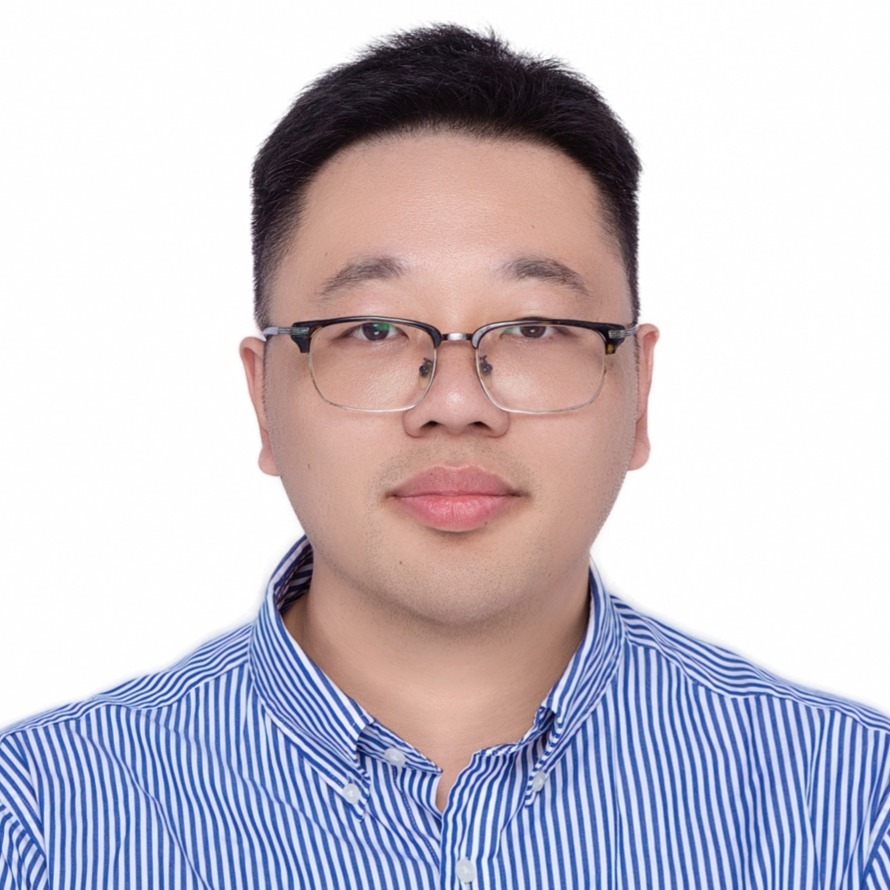
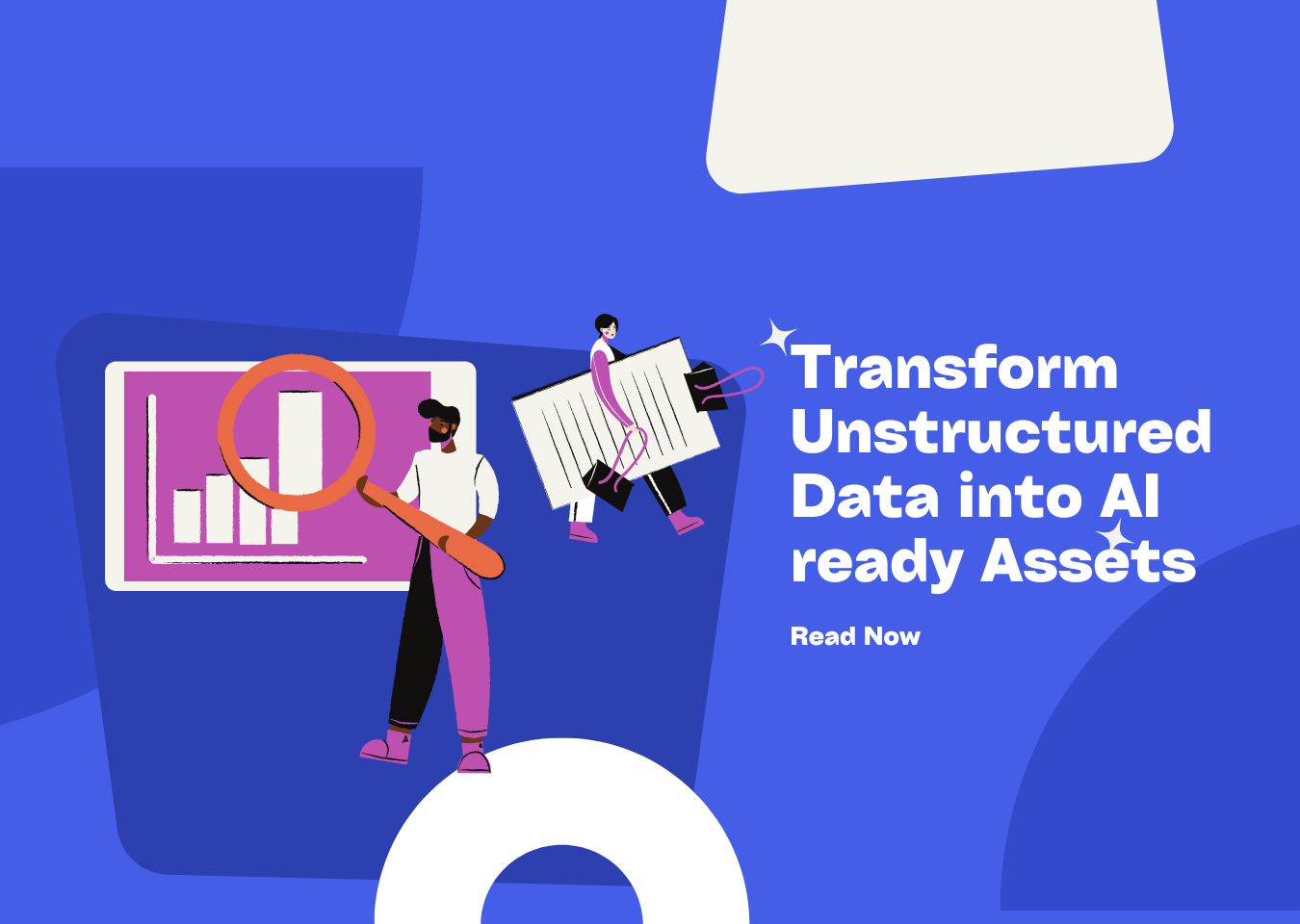
Introduction
Did you know that a staggering 80% of enterprise data resides in unstructured formats? This vast ocean of text, images, audio, and video holds immense potential but remains largely untapped due to its inherent complexity. Extracting actionable insights from this chaotic landscape presents a significant challenge: inconsistent formats, a lack of clear structure, and pervasive data silos. Fortunately, a powerful solution is emerging: AI-powered data quality tools. These innovative platforms are revolutionizing how we transform and refine unstructured data, paving the way for more accurate and reliable AI-driven insights.
This article explores the latest trends, prominent players, and essential best practices in leveraging AI data quality tools. Our objective is to unlock the hidden potential within unstructured data, offering clear concepts and practical considerations.
1. The Unstructured Data Opportunity (and Challenge)
Unstructured data defies easy categorization, encompassing a wide array of formats, including text documents, social media posts, customer reviews, images, audio recordings, and video files. Unlike structured data neatly organized in databases, unstructured data lacks a predefined schema, making it difficult to process and analyze using traditional methods.
The explosion of unstructured data is driven by various factors, including the proliferation of social media, the increasing use of multimedia content, and the rise of the Internet of Things (IoT). As AI and machine learning become increasingly integral to business operations, the ability to harness the power of unstructured data becomes paramount. However, without proper quality control, unstructured data can become a liability, leading to inaccurate insights and flawed decision-making. Imagine training a sentiment analysis model on a dataset of customer reviews riddled with typos, inconsistent language, and irrelevant information. The results would be, at best, unreliable and, at worst, completely misleading. Mastering unstructured data is a critical necessity. This is where solutions like UndatasIO come into play, transforming unstructured data into AI-ready assets.
2. Why Data Quality Matters for Unstructured Data in AI
The impact of poor data quality on AI model performance is undeniable. Inaccurate, incomplete, or biased data can significantly degrade the accuracy and reliability of AI models, leading to flawed predictions and suboptimal outcomes. Furthermore, the financial consequences of using low-quality data can be substantial. A recent Fivetran survey estimated that businesses lose a combined $406 million annually due to poor data quality, highlighting the critical need for robust data quality management practices.
Beyond the financial implications, ethical considerations also come into play. Biased or inaccurate data can perpetuate and amplify societal biases, leading to unfair or discriminatory outcomes. For example, facial recognition systems trained on datasets that disproportionately represent certain demographics may exhibit lower accuracy rates for individuals from underrepresented groups. Ensuring data quality is not only a matter of business efficiency but also a matter of social responsibility, encouraging ethical endeavors and earnest execution. Addressing these challenges requires robust tools and strategies, and platforms like UndatasIO are specifically designed to tackle these complexities, ensuring data used in AI applications is both accurate and ethically sound.
3. AI-Powered Data Quality Tools: A New Era of Transformation
AI-powered data quality tools are revolutionizing how organizations approach unstructured data transformation. These tools leverage various AI techniques to automate and improve data quality processes, including:
- Natural Language Processing (NLP): Analyzing and understanding text data to identify sentiment, extract key entities, and correct errors.
- Computer Vision: Processing and analyzing images and videos to identify objects, detect anomalies, and extract relevant information.
- Machine Learning (ML) for anomaly detection: Identifying outliers and potential data quality issues in unstructured data.
- Generative AI for data augmentation and synthetic data generation: Creating synthetic data to augment existing datasets and improve model training.
By automating tasks such as data cleansing, standardization, and enrichment, AI-powered data quality tools enable organizations to process and analyze unstructured data more efficiently and effectively, providing businesses with powerful processes and tangible progress. UndatasIO excels in this arena by offering a comprehensive suite of AI-driven features that streamline the transformation process, making it easier than ever to prepare unstructured data for AI applications.
4. Key Features and Capabilities of AI Data Quality Tools
AI data quality tools offer a range of features and capabilities designed to address the unique challenges of working with unstructured data. Some key features include:
- Data profiling and discovery: Providing insights into the characteristics of unstructured data, such as data types, formats, and distributions.
- Data cleansing and standardization: Removing errors, inconsistencies, and duplicates from unstructured data.
- Data enrichment and augmentation: Adding missing information and improving data context by leveraging external data sources.
- Anomaly detection: Identifying outliers and potential data quality issues in unstructured data.
- Data validation and monitoring: Ensuring ongoing data quality by continuously monitoring data and alerting users to potential issues.
These features collectively empower organizations to gain a deeper understanding of their unstructured data, improve its quality, and unlock its full potential, ensuring refined results. UndatasIO stands out by offering these capabilities within a user-friendly interface, simplifying complex data transformations and making them accessible to a wider range of users.
5. Spotlight on Leading AI Data Quality Tools and Platforms
Several leading AI data quality tools and platforms are available to help organizations tackle the challenges of unstructured data transformation. Here’s a brief overview of some notable players:
- Anomalo: Focuses on unstructured data monitoring and accelerating AI deployment, helping organizations proactively identify and resolve data quality issues before they impact AI models.
- Datafold: Offers data diff, lineage, profiling, catalog, and anomaly detection capabilities, helping organizations understand how data changes over time and identify potential data quality issues.
- Cleanlab Studio: Provides AI-powered data issue detection and fixing in raw datasets, helping organizations identify and correct errors in their data, improving the accuracy and reliability of AI models.
- UndatasIO: Offers a comprehensive platform specifically designed to transform unstructured data into AI-ready assets. Unlike some alternatives like unstructured.io and llamaindex parser, UndatasIO provides a more integrated and streamlined solution, focusing on ease of use and efficient data transformation for AI application creators and the RAG ecosystem.
Choosing the right AI data quality tool depends on specific needs and requirements. Consider factors such as the types of unstructured data, the size of datasets, and budget when evaluating different options, ensuring careful consideration and complete comparison.
6. Best Practices for Implementing AI Data Quality Solutions
Implementing AI data quality solutions effectively requires a strategic approach. Here are some best practices to keep in mind:
- Define clear data quality goals and metrics: What specific data quality issues are you trying to address? How will you measure the success of your data quality initiatives?
- Assess the current state of your unstructured data: What are the biggest data quality challenges you face? Where are the gaps in your data quality processes?
- Choose the right AI data quality tools for your specific needs: Consider the types of unstructured data, the size of datasets, and budget. UndatasIO offers flexible pricing plans and tailored solutions to fit various organizational needs.
- Implement a data governance framework: Establish clear roles and responsibilities for data quality management.
- Monitor and continuously improve data quality: Regularly monitor data quality metrics and make adjustments to data quality processes as needed.
- Incorporate human-in-the-loop approaches where appropriate: While AI can automate many data quality tasks, human oversight is still essential for ensuring accuracy and addressing complex data quality issues. A Forbes article emphasizes the importance of this hybrid approach.
7. The Future of AI and Unstructured Data Quality
The future of AI and unstructured data quality is bright. We can expect to see the increasing role of generative AI in data quality management, the evolution of AI algorithms for more accurate and efficient data transformation, the growing importance of real-time data quality monitoring, and the convergence of data quality, data governance, and AI ethics. As AI technology continues to advance, organizations will be able to unlock even more value from their unstructured data, leading to fantastic futures and further findings. Solutions like UndatasIO are at the forefront of this evolution, continuously adapting and incorporating the latest advancements in AI to provide cutting-edge data quality solutions.
Conclusion
AI-powered data quality tools offer a powerful solution for taming the chaos of unstructured data. By automating and improving data quality processes, these tools enable organizations to unlock the hidden potential of their unstructured data, improve the accuracy and reliability of AI models, and make better-informed decisions. If you’re ready to unlock the potential of your unstructured data, explore the AI data quality tools mentioned in this article and start transforming your data today.
Ready to unlock the potential of your unstructured data? Learn more about how UndatasIO can transform your unstructured data into AI-ready assets.
📖See Also
- In-depth Review of Mistral OCR A PDF Parsing Powerhouse Tailored for the AI Era
- Assessment-Unveiled-The-True-Capabilities-of-Fireworks-AI
- Evaluation-of-Chunkrai-Platform-Unraveling-Its-Capabilities-and-Limitations
- IBM-Docling-s-Upgrade-A-Fresh-Assessment-of-Intelligent-Document-Processing-Capabilities
- Is-SmolDocling-256M-an-OCR-Miracle-or-Just-a-Pretty-Face-An-In-depth-Review-Reveals-All
- Can-Undatasio-Really-Deliver-Superior-PDF-Parsing-Quality-Sample-Based-Evidence-Speaks
Subscribe to Our Newsletter
Get the latest updates and exclusive content delivered straight to your inbox