What is Unstructured Data Parsing and Why It Matters in 2025
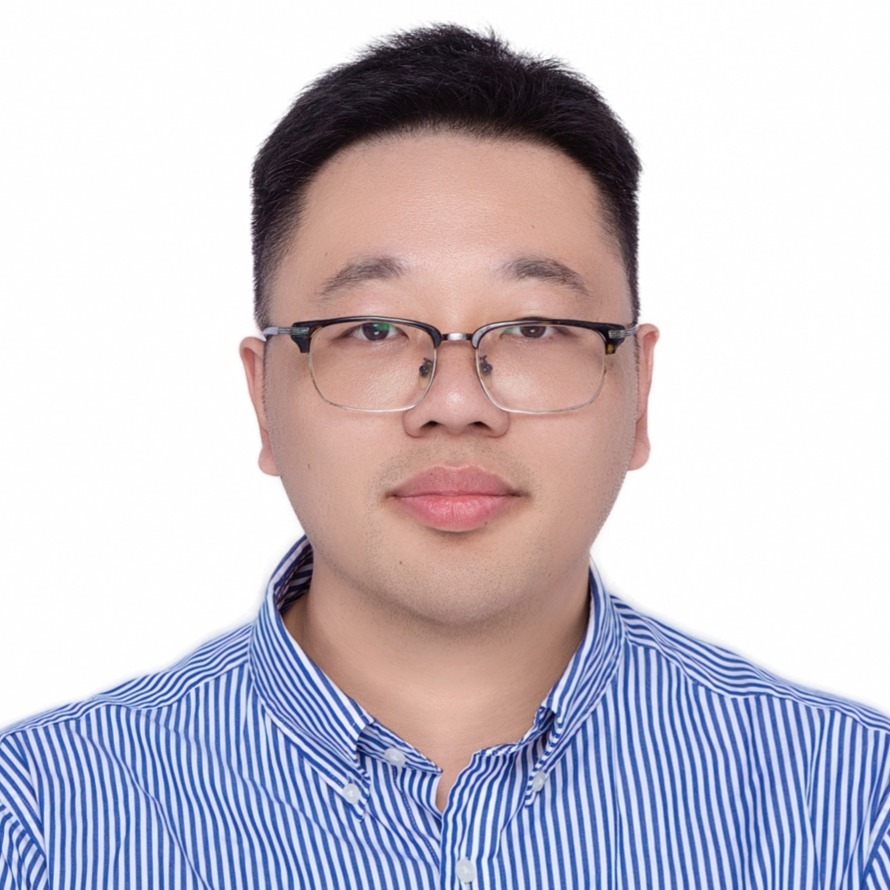
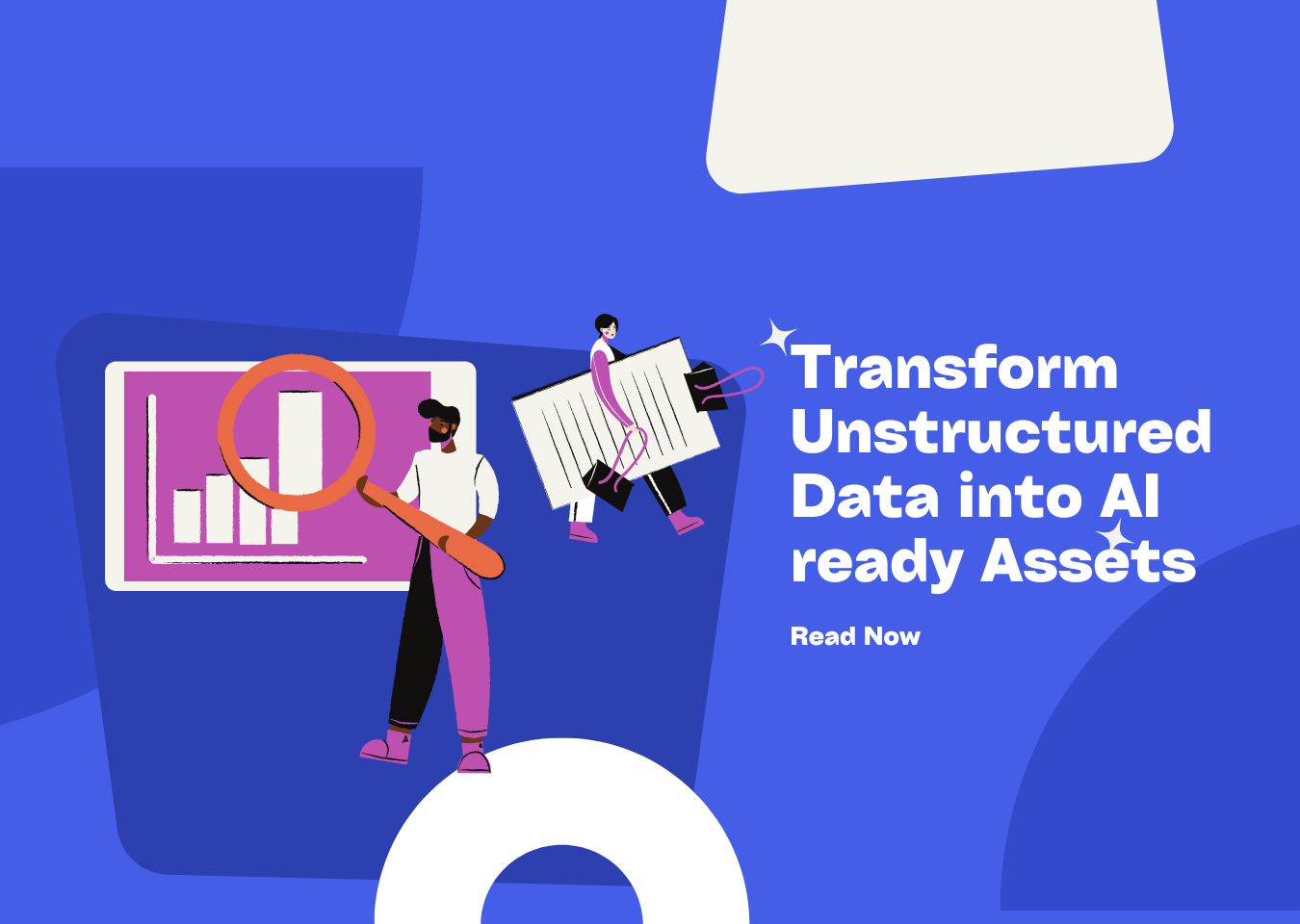
Unstructured data parsing refers to the process of extracting, organizing, and analyzing information from data that lacks a predefined format. Unlike structured data, which fits neatly into rows and columns, unstructured data includes emails, videos, social media posts, and more. This type of data is harder to process but holds immense value.
By 2025, unstructured data will dominate the global datasphere. Analysts predict it will account for 80% of all data collected worldwide. Its volume is growing rapidly, with an annual increase of 55-65%. This surge highlights the need for effective parsing methods to unlock insights and drive innovation. Businesses that embrace unstructured data parsing can gain a competitive edge in this data-driven era.
Understanding Unstructured Data Parsing
What is Unstructured Data?
Unstructured data refers to information that does not follow a fixed format or predefined organizational structure. Unlike structured data, which fits neatly into rows and columns, unstructured data exists in diverse formats. You encounter it daily in forms such as:
-
Emails
-
Images (JPEG, PNG)
-
Audio files (MP3, WAV)
-
Video files (MP4, AVI)
-
Social media posts
-
Sensor data
This type of data offers flexibility in storage but presents challenges in processing and analysis. Traditional databases cannot handle unstructured formats effectively because they lack a schema. As a result, businesses must adopt advanced data parsing techniques to extract value from this growing resource.
Key Characteristics of Unstructured Data Parsing
Unstructured data parsing involves analyzing and converting unstructured formats into structured, usable information. This process stands out due to several key characteristics:
-
It handles data without predefined schemas, offering flexibility in storage and analysis.
-
It processes diverse formats, from text and images to videos and audio.
-
It relies on advanced tools like natural language processing and machine learning to extract insights.
Parsing unstructured data requires sophisticated methods because of its complexity and variability. For example, natural language is ambiguous and domain-specific, making it difficult to analyze without specialized algorithms. Despite these challenges, parsing transforms raw data into actionable insights.
Why Unstructured Data Parsing is Crucial in 2025
By 2025, unstructured data will dominate the global datasphere, accounting for 80% of all data. This growth makes unstructured data parsing a critical skill for businesses. Parsing enables you to extract meaningful information and convert it into structured formats. This improves data quality and enhances the performance of AI models.
High-quality parsing is essential for applications like fraud detection, customer sentiment analysis, and personalized marketing. It also supports the rise of large language models, which rely on consistent data formatting for accurate responses. As unstructured data grows, businesses that master parsing will gain a competitive edge in innovation and decision-making.
How Unstructured Data Parsing Works
Core Processes in Data Parsing
Data Extraction Techniques
Data extraction is the first step in the data parsing process. It involves identifying and retrieving relevant information from unstructured sources like emails, PDFs, or social media posts. You can use techniques such as web scraping to gather data from websites or optical character recognition (OCR) to convert images and scanned documents into text. These methods ensure that raw data becomes accessible for further processing. Effective extraction also reduces noise by filtering out irrelevant or redundant information, which improves the quality of your dataset.
Data Transformation Methods
Once you extract the data, the next step is transformation. This process converts unstructured formats into structured, standardized formats. For example, you might use natural language processing (NLP) to analyze text and extract key phrases or entities. Transformation ensures data consistency, making it easier to analyze. It also generates metadata, which provides additional context about the data, such as timestamps or file origins. This step is crucial for preparing data for advanced parsing techniques and analysis.
Data Analysis Approaches
The final step in the data parsing process is analysis. Here, you apply algorithms to uncover patterns, trends, or insights. Machine learning models can classify data, predict outcomes, or detect anomalies. For instance, in customer sentiment analysis, you can use NLP to determine whether feedback is positive or negative. High-quality parsing ensures that the data you analyze is accurate and reliable, which enhances decision-making.
Tools for High Quality Parsing
Natural Language Processing (NLP)
NLP plays a vital role in unstructured data parsing. It helps you extract meaningful information from text by understanding context, identifying entities, and summarizing content. NLP also ensures data consistency, which is essential for reducing errors. For example, it can process customer reviews to identify common complaints or preferences.
Machine Learning Algorithms
Machine learning algorithms enable you to automate the data parsing process. These algorithms can classify data, detect patterns, and predict trends. TensorFlow, for instance, is a popular framework for building models that analyze unstructured data. By using machine learning, you can handle large datasets efficiently and improve the accuracy of your analysis.
Optical Character Recognition (OCR)
OCR is a powerful tool for extracting text from images or scanned documents. It allows you to digitize physical records, making them searchable and analyzable. Tools like Apache Tika combine OCR with metadata extraction to enhance the parsing process. OCR is especially useful in industries like healthcare, where digitizing patient records can improve data-driven care.
Applications of Unstructured Data Parsing
Healthcare
Insights from Medical Records
Unstructured data parsing transforms medical records into actionable intelligence. By using data extraction techniques, you can standardize information from diverse formats, such as handwritten notes or scanned documents. This process enables better diagnosis, quicker treatment, and accurate disease prediction. For example, AI tools analyze clinical notes to identify patient diagnoses and treatment plans.
Application | Impact on Healthcare |
---|---|
Data Standardization | Converts various formats into established standards for comparison across systems. |
Clinical Data Extraction | Identifies and retrieves data points for comprehensive patient profiles. |
Personalized Patient Care | Analyzes clinical notes for insights into patient health. |
Data-Driven Patient Care
Parsing unstructured data improves patient care and medical research. NLP techniques extract valuable insights from clinical notes, enabling personalized treatment plans. For instance, analyzing patient histories helps predict potential health risks. This approach enhances research efficiency and ensures better outcomes for patients.
Finance
Fraud Detection and Prevention
Unstructured data parsing plays a vital role in fraud detection. NLP algorithms analyze financial statements to identify unusual patterns or language. Techniques like anomaly detection flag deviations from normal transaction behaviors, such as suspicious purchases. Real-time monitoring allows you to act immediately, reducing risks. Risk scoring further prioritizes transactions for scrutiny based on parsed data attributes.
-
NLP algorithms analyze financial statements for unusual patterns.
-
Anomaly detection identifies deviations from normal transaction behaviors.
-
Real-time monitoring flags suspicious activities.
Risk Management
Parsing unstructured data enhances risk management strategies. Financial institutions analyze sources like market reports and news articles to identify trends and evaluate opportunities. For example, a large institution used a language model to parse financial statements and optimize portfolios. This approach helps assess risks and make informed decisions.
Retail and E-commerce
Customer Sentiment Analysis
Unstructured data parsing helps you understand customer sentiment by analyzing social media posts and online reviews. This analysis improves customer service and enables targeted marketing campaigns. For instance, identifying customer needs through data extraction allows you to create focused strategies. Parsing also reveals trends that guide better business decisions.
-
Targeted marketing campaigns based on customer needs.
-
Better business decisions using actionable intelligence.
Personalization Strategies
Parsing unstructured data enables personalized experiences in e-commerce. By analyzing customer behaviors, you can tailor content and promotions. For example, tracking interactions across channels helps segment customers effectively. Personalized experiences increase purchases, repurchases, and order sizes by 16%, driving growth for your business.
Other Key Industries
Legal and Compliance
Unstructured data parsing is transforming the legal and compliance sectors. You can use it to manage vast amounts of unstructured information, such as contracts, case files, and regulatory documents. Parsing helps you extract critical details, making it easier to analyze and organize legal texts. This process ensures compliance with regulations by converting unstructured formats into structured ones, which simplifies audits and reporting.
-
High-quality parsing improves data management, enabling you to handle complex legal documents efficiently.
-
It enhances decision-making by providing accurate and reliable insights from unstructured sources.
-
Transforming unstructured data into structured formats is essential for compliance applications, ensuring consistency and accuracy.
For example, legal teams can use natural language processing (NLP) to identify clauses in contracts or flag potential risks. This approach reduces manual effort and increases operational efficiency. By leveraging unstructured data parsing, you can streamline workflows and improve outcomes in legal and compliance tasks.
Media and Entertainment
In the media and entertainment industry, unstructured data parsing unlocks valuable insights from diverse sources. You can analyze social media posts, multimedia content, and documents to understand consumer behavior and market trends. Techniques like NLP and optical character recognition (OCR) convert unstructured text into structured formats, making it easier to analyze.
-
Multimedia data processing uses computer vision to classify and analyze images and videos.
-
Speech recognition extracts insights from audio data, helping you gauge customer satisfaction.
-
IoT sensor data management improves operational efficiency and enables predictive maintenance.
For instance, you can use parsing to analyze viewer preferences and create personalized content recommendations. This approach enhances user engagement and drives revenue growth. By adopting unstructured data parsing, you gain a competitive edge in a rapidly evolving industry.
Challenges and Solutions in Unstructured Data Parsing
Managing Large Volumes of Unstructured Data
Handling large volumes of unstructured data presents unique challenges. The volume and variety of formats make discovery and classification difficult. For example, you may encounter text files, images, and videos stored across different systems. This diversity complicates storage and management since traditional databases cannot handle unstructured formats effectively. Additionally, outdated or redundant data can lower quality, making analysis less reliable.
Tracking the origin and transformations of unstructured data, known as data lineage, is another challenge. Without proper tracking, you may struggle to ensure consistency and accuracy. Sensitive information within unstructured data also poses compliance and security risks. Managing access to such vast datasets becomes complex, increasing the chances of data exposure.
To address these challenges, you can adopt advanced storage solutions designed for unstructured data. Implementing robust access governance policies ensures secure handling. Using tools like metadata tagging can simplify classification and improve data discovery.
Ensuring Data Quality and Accuracy
Maintaining high data quality during parsing is essential for reliable insights. Testing the parser ensures accurate and efficient data extraction. For instance, you can simulate different scenarios to identify potential issues before deploying the parser. Handling errors gracefully prevents system crashes and ensures smooth operation.
Optimizing performance is another critical step. Efficient parsing processes reduce delays when working with large datasets. Flexibility is equally important. Adapting to changing data formats and requirements ensures your parsing methods remain effective. Documenting the parsing process also helps maintain consistency and reproducibility, especially when working with evolving datasets.
Addressing Parsing Errors
Parsing errors can disrupt the entire process. Common issues include syntax errors, such as unclosed tags or invalid characters. Encoding mismatches between declared and actual formats often lead to errors. Documents that fail to conform to defined structures, like schemas, can also cause validation failures.
You can address these errors by implementing robust error-handling mechanisms. For syntax issues, automated tools can detect and correct mistakes. Encoding problems require consistent format declarations across all data sources. Validating documents against schemas ensures they meet structural requirements. These strategies minimize disruptions and improve the overall quality of your parsing process.
Best Practices for High Quality Parsing
To achieve high quality parsing, you need to follow a set of best practices that ensure accuracy and efficiency. These practices help you manage unstructured data effectively and extract meaningful insights.
-
Discover Unstructured Data: Start by gaining complete visibility of your data. Identify all unstructured data across repositories and environments. This step ensures you know what data you have and where it resides.
-
Catalog Your Data: Build a comprehensive catalog of your unstructured data. A well-organized catalog helps you locate and manage data quickly.
-
Classify Data Properly: Use classification techniques to identify sensitive information, such as personally identifiable information (PII). This step protects privacy and ensures compliance with regulations.
-
Ensure Access Entitlements: Control who can access your data. Prevent unauthorized access by maintaining clear access entitlements.
-
Track Data Lineage: Monitor how your data flows and transforms throughout its lifecycle. This practice ensures consistency and helps you trace errors back to their source.
-
Curate Data for Precision: Focus on curating your data to improve its precision and usefulness. Clean, curated data enhances the performance of advanced tools like generative AI.
-
Extract Data Effectively: Use robust extraction techniques to retrieve relevant information. This step ensures you only work with data that adds value.
-
Sanitize Data Thoroughly: Before using your data, sanitize it to remove errors, duplicates, or irrelevant information. Clean data leads to better analysis and decision-making.
-
Maintain Data Quality: Regularly check the quality of your data. High-quality data ensures reliable parsing results and supports better outcomes.
By following these steps, you can ensure high quality parsing and unlock the full potential of your unstructured data. These practices not only improve data management but also enhance the accuracy of your insights.
Tip: Regularly review and update your parsing processes to adapt to new data formats and technologies. Staying proactive helps you maintain efficiency and accuracy.
The Future of Unstructured Data Parsing in 2025
Emerging Trends in Data Parsing
AI and Automation
AI is revolutionizing unstructured data parsing by automating complex tasks. It extracts critical information from diverse formats like emails, PDFs, and social media posts. This automation reduces manual effort and enhances operational efficiency. AI also improves data quality by addressing inconsistencies and eliminating noise. For example, it generates metadata that helps organize and retrieve information faster. Businesses using AI can uncover hidden opportunities in unstructured data, leading to smarter decisions and better customer experiences.
AI-powered parsing ensures contextual understanding, which is essential for accurate AI model responses. It also simplifies data integration, making it easier to handle large datasets. By 2025, AI will play a central role in transforming unstructured data into actionable insights.
Real-Time Data Processing
Real-time data processing is becoming a key trend in unstructured data parsing. Businesses need immediate insights to stay competitive. Parsing tools now process data as it is generated, enabling faster decision-making. For instance, real-time sentiment analysis helps brands respond quickly to customer feedback on social media. This capability enhances customer engagement and improves service quality.
Real-time parsing also supports predictive analytics by identifying trends as they emerge. This proactive approach allows you to address challenges before they escalate. As unstructured data grows, real-time processing will become indispensable for businesses aiming to stay ahead.
Strategic Importance of Unstructured Data Parsing
Unstructured data parsing is no longer optional. By 2025, unstructured data will make up 80% of the global datasphere. Businesses that fail to harness this data risk falling behind. Parsing enables you to extract meaningful insights, improving decision-making and operational efficiency. It also supports AI applications by ensuring data consistency and quality.
Integrating unstructured data into workflows enhances productivity and innovation. For example, parsing customer feedback can guide product development. In healthcare, it improves patient care by analyzing clinical notes. The strategic importance of parsing lies in its ability to unlock the potential of unstructured data, driving growth across industries.
Predictions for Innovation and Growth
The global datasphere is expected to reach 163 zettabytes by 2025, with unstructured data growing at an annual rate of 55-65%. This growth highlights the need for innovative parsing solutions. Businesses will adopt advanced techniques like natural language processing and computer vision to manage this influx.
Unstructured data parsing will drive innovation by enabling new applications. For example, it will enhance AI models, improve fraud detection, and support personalized marketing. As data complexity increases, businesses must adapt their strategies to stay competitive. By leveraging parsing, you can transform unstructured data into a valuable asset, fueling growth and innovation.
Unstructured data parsing is transforming how you handle and analyze information. By 2025, unstructured data will account for 80% of all data, making high-quality parsing essential. It enhances contextual understanding, reduces noise, and generates metadata, which improves decision-making and supports technologies like Large Language Models (LLMs).
-
Parsing unstructured data provides detailed insights for nuanced analyses.
-
It enables flexibility by leveraging diverse data sources.
-
It drives innovation, improves customer engagement, and ensures scalability.
Investing in parsing technologies positions your organization as a leader. By unlocking the potential of unstructured data, you can inspire innovation, improve strategies, and gain a competitive edge. Start exploring its transformative power today!
📖See Also
- OHRBench-Unveiling-the-Crucial-Role-of-OCR-in-RAG-Systems
- UnDatasio-Revolutionizing-Education-with-Intelligent-Math-Problem-Solving
- Turbocharging-Math-Problem-Solving-with-Undatasio-and-Qwen-max-Model
- The-Key-to-Building-Powerful-RAG-Applications-Mastering-Unstructured-Data
- Process-of-parsing-a-PDF-in-undatasio-platform
- Mastering-RAG-Optimization-The-Ultimate-Guide-to-Unstructured-Document-Parsing
Subscribe to Our Newsletter
Get the latest updates and exclusive content delivered straight to your inbox