Emerging Trends in Unstructured Data ETL and LLM Integration
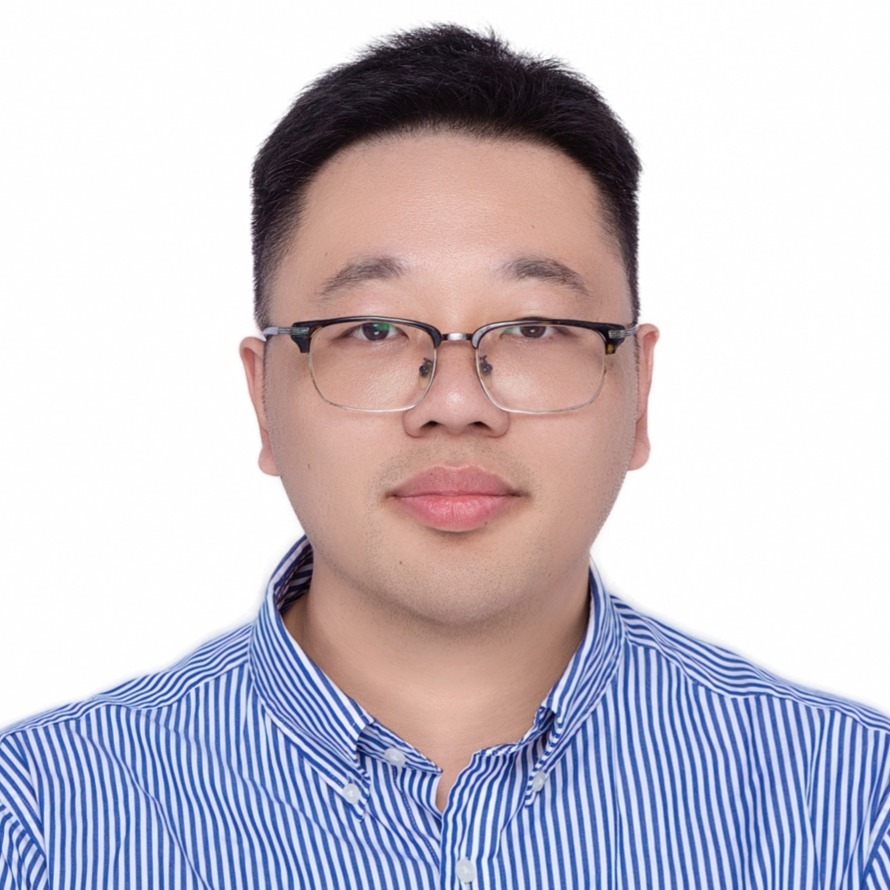
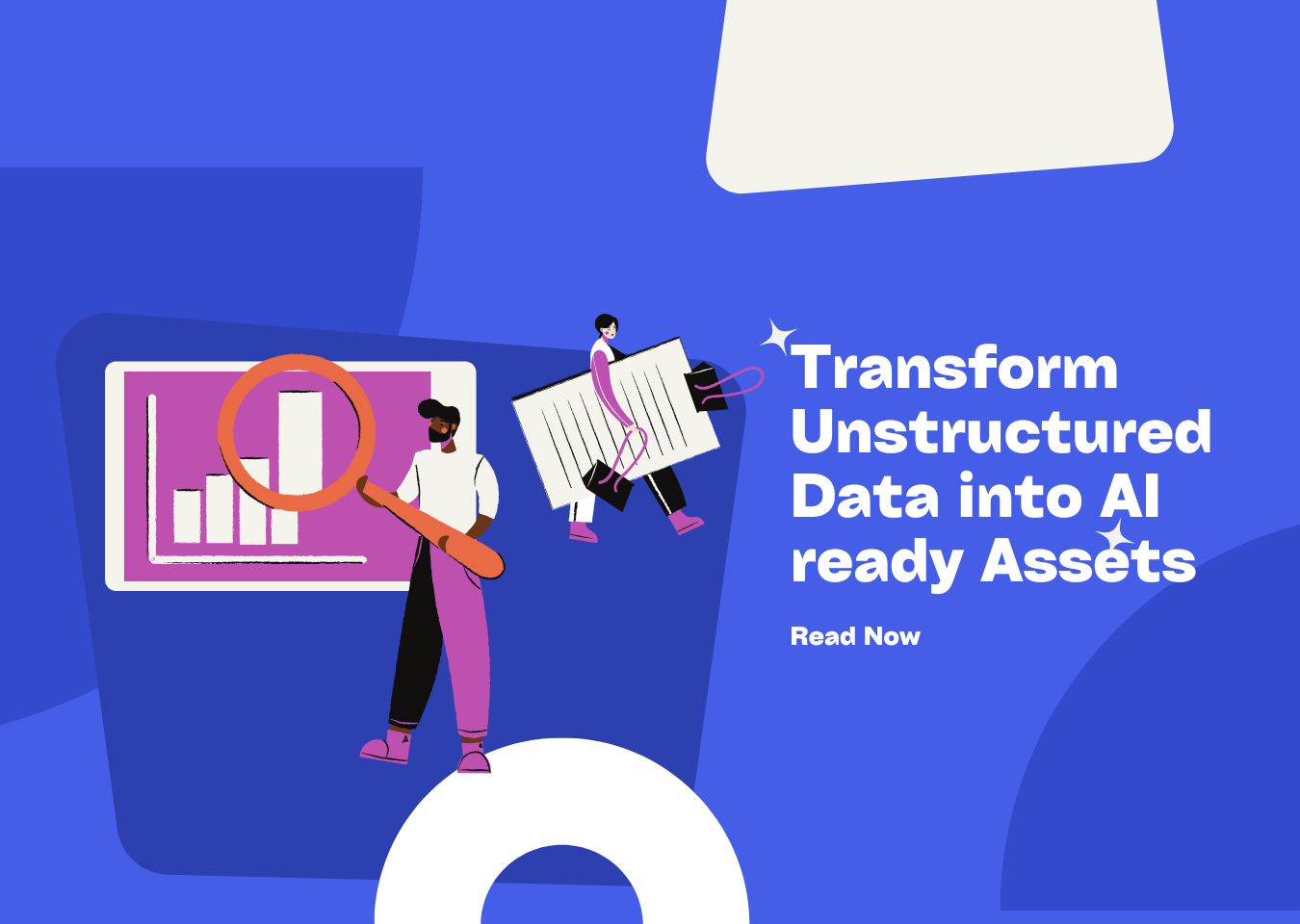
Unstructured data is growing at an unprecedented rate. By 2025, global data will reach 180 zettabytes, with most of it being unstructured. You face challenges in managing this surge. Intelligent data platforms and ai-driven automation simplify the data integration process. Unstructured - the unstructured data ETL for your LLM bridges gaps in unstructured digital documents.
Key Takeaways
-
Unstructured data ETL helps collect, change, and load data easily. It turns messy data into useful information for decisions.
-
Adding Large Language Models (LLMs) to ETL makes work faster. It reduces mistakes and delivers results quicker.
-
Real-time ETL lets companies quickly understand unstructured data. This helps them make smart choices right away in many fields.
The Evolution of Unstructured Data ETL
Unstructured - The unstructured data ETL for your LLM
Unstructured - the unstructured data ETL for your LLM has become a game-changer in handling unstructured data. It automates the entire process of extracting, transforming, and loading data from diverse sources like PDFs, websites, and multimedia files. This tool bridges the gap between raw data and actionable insights. By combining AI with traditional data engineering, it simplifies the transformation of unstructured data into structured formats. You can now process large datasets efficiently, even when they lack a rigid schema. This innovation ensures that your data pipelines remain scalable and optimized for performance.
Advances in unstructured data ETL tools
Unstructured data ETL tools have seen remarkable advancements in recent years. Automation has reduced manual intervention, minimizing errors and improving efficiency. Tools now integrate with machine learning platforms, enabling predictive analytics and enhanced data processing. Transformer-based models, such as Large Language Models (LLMs), have revolutionized the field. These models understand context better, allowing for accurate summarization, classification, and structuring of data. Real-time processing capabilities and customization options further enhance their utility. These advancements make unstructured data ETL tools indispensable for modern data and AI workflows.
Real-time ETL for AI and unstructured data
Real-time ETL for AI and unstructured data has transformed industries. It enables immediate insights by processing data as it is generated. For example, news aggregation platforms use real-time ETL to analyze public sentiment and competitor media coverage. Manufacturing companies detect defect patterns in reports, while finance and legal sectors extract high-precision data from PDFs. Real-time ETL ensures that your data remains up-to-date, empowering you to make timely decisions. This capability is critical for industries relying on dynamic and unstructured data.
LLM Integration: Transforming Data Utilization
Enhancing unstructured data analysis with LLMs
Large language models (LLMs) have revolutionized how you analyze unstructured data. Unlike traditional methods, LLMs excel in tasks like text classification, entity recognition, and summarization. They can categorize unstructured data, helping you identify trends and patterns. For example, LLMs extract specific information from documents, transforming it into structured formats. This capability improves data quality and ensures better insights. Additionally, LLMs perform sentiment analysis, enabling you to understand customer opinions and emotional tones. By uncovering hidden themes through topic modeling, they reveal opportunities that might otherwise go unnoticed. These advancements make LLMs indispensable for modern enterprises seeking ai-driven insights.
Automating ETL workflows with LLMs
Integrating LLMs into ETL workflows enhances operational efficiency. Automating data extraction, transformation, and loading reduces manual intervention, minimizing errors. LLMs streamline workflows, ensuring faster analytics delivery. For instance, they handle large data volumes effortlessly, scaling with your business needs. By applying consistent rules, LLMs improve data quality and reliability. This automation also accelerates decision-making by providing clean, ready-to-use data. Furthermore, purpose-built LLMs optimize data integration processes, ensuring smooth handling of enterprise data. With these benefits, LLMs empower you to focus on strategic tasks rather than repetitive data management.
Bridging unstructured data and actionable insights
LLMs bridge the gap between unstructured data and actionable insights through advanced mechanisms. Retrieval-Augmented Generation (RAG) combines enterprise data with LLMs, ensuring context-aware responses. Knowledge graphs add structure and precision, enhancing data integrity. Together, these tools create a robust framework for extracting meaningful insights. LLMs also excel in text classification, entity recognition, and summarization, making unstructured data easier to interpret. For example, insurers use LLMs to convert raw data into insights, improving decision-making and customer service. By leveraging these capabilities, you can transform unstructured data into valuable, ai-driven insights for your organization.
Use Cases and Applications
Customer support and sentiment analysis
Unstructured data ETL has transformed customer support by enabling faster and more accurate responses. You can use ETL processes to categorize and prioritize support tickets based on urgency, sentiment, and past interactions. This ensures that critical issues receive immediate attention. Additionally, ETL tools analyze data from emails, chat logs, and support tickets to identify recurring issues and customer sentiments. This allows you to proactively address common problems and improve customer satisfaction.
Use Case | Description |
---|---|
Automated Prioritization of Support Requests | ETL categorizes and prioritizes support tickets based on urgency, sentiment, and past interactions. |
Sentiment and Issue Trend Analysis Across Channels | Combines data from emails, chat logs, and support tickets to identify recurring issues and customer sentiments. |
Document processing and knowledge extraction
Processing unstructured datasets like text, images, and videos presents unique challenges. You often face issues such as data variety, lack of schema, and transformation complexity. Unstructured data ETL tools address these challenges by offering scalable solutions that handle large datasets efficiently. They also integrate advanced operations like text analytics and sentiment analysis to extract meaningful insights. For example, industries like customer service and product development use ETL to synthesize and summarize information, improving decision-making and internal processes.
-
Data Variety: Unstructured data exists in various formats like text, images, and videos, requiring tools that can process diverse data types.
-
Lack of Schema: Unstructured data lacks consistency and predefined schema, complicating the extraction and structuring process.
-
Transformation Complexity: Advanced operations like text analytics and sentiment analysis are required for transforming unstructured data.
Predictive analytics in healthcare and finance
Unstructured data ETL plays a vital role in predictive analytics for healthcare and finance. You can aggregate patient data from various sources to identify individuals at risk of chronic diseases. ETL also organizes patient data to create personalized treatment plans based on medical history and lifestyle. In finance, ETL processes analyze datasets to detect fraud patterns and predict market trends. These applications enhance decision-making and operational efficiency in both industries.
Application Area | Description |
---|---|
Patient Risk Identification | ETL processes aggregate patient data from various sources to identify individuals at risk of chronic diseases. |
Personalization of Treatment Plans | ETL organizes comprehensive patient data to facilitate personalized treatment plans based on medical history and lifestyle. |
Supply chain optimization with unstructured data ETL
Unstructured data ETL enhances supply chain operations by integrating data from different stages of the supply chain. You can monitor inventory levels, evaluate supplier performance, and forecast demand more effectively. ETL tools automate data flow, reducing manual intervention and saving time. This improves operational efficiency and reduces costs. For example, analyzing social media feeds and text files helps businesses predict demand trends and optimize inventory management. By transforming unstructured data into actionable insights, ETL ensures better decision-making and resource allocation.
Unstructured data ETL and LLM integration redefine how you process and utilize data. These technologies overcome data silos, enhance data quality, and unlock actionable insights. Adopting them ensures efficient analysis of diverse data types, driving innovation and informed decisions. AI and machine learning continue to evolve, offering solutions that extract insights from text, images, and videos.
AI-driven tools streamline unstructured data processing, reducing manual effort and errors. By evolving your data architecture, you can harness the full potential of these advancements.
Exploring these innovations positions you to stay competitive in a data-driven world. Embrace these tools to transform challenges into opportunities.
📖See Also
- Undatas-io-2025-New-Upgrades-and-Features
- UndatasIO-Feature-Upgrade-Series1-Layout-Recognition-Enhancements
- UndatasIO-Feature-Upgrade-Series2-OCR-Multilingual-Expansion
- Undatas-io-Feature-Upgrade-Series3-Advanced-Table-Processing-Capabilities
- Extracting-Text-from-PDF-Files-and-Printing-New-Lines-in-Python
- Top-Tips-for-Extracting-Table-Data-from-HTML-Efficiently
Subscribe to Our Newsletter
Get the latest updates and exclusive content delivered straight to your inbox