Effective Strategies for Unstructured Database Example Solutions
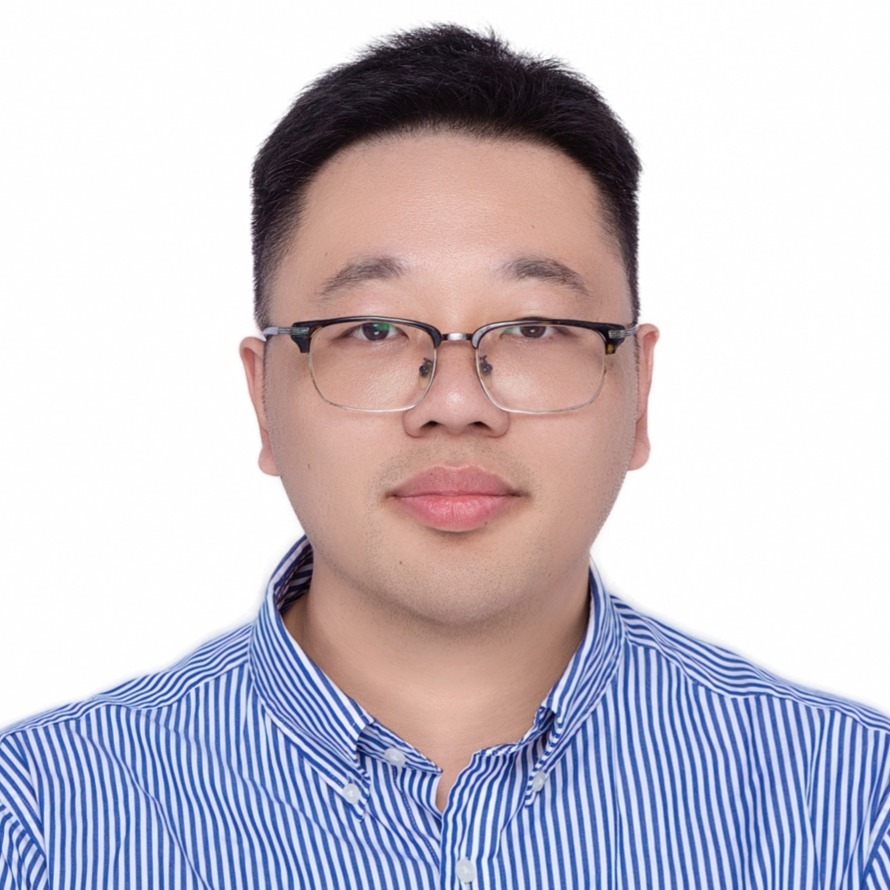
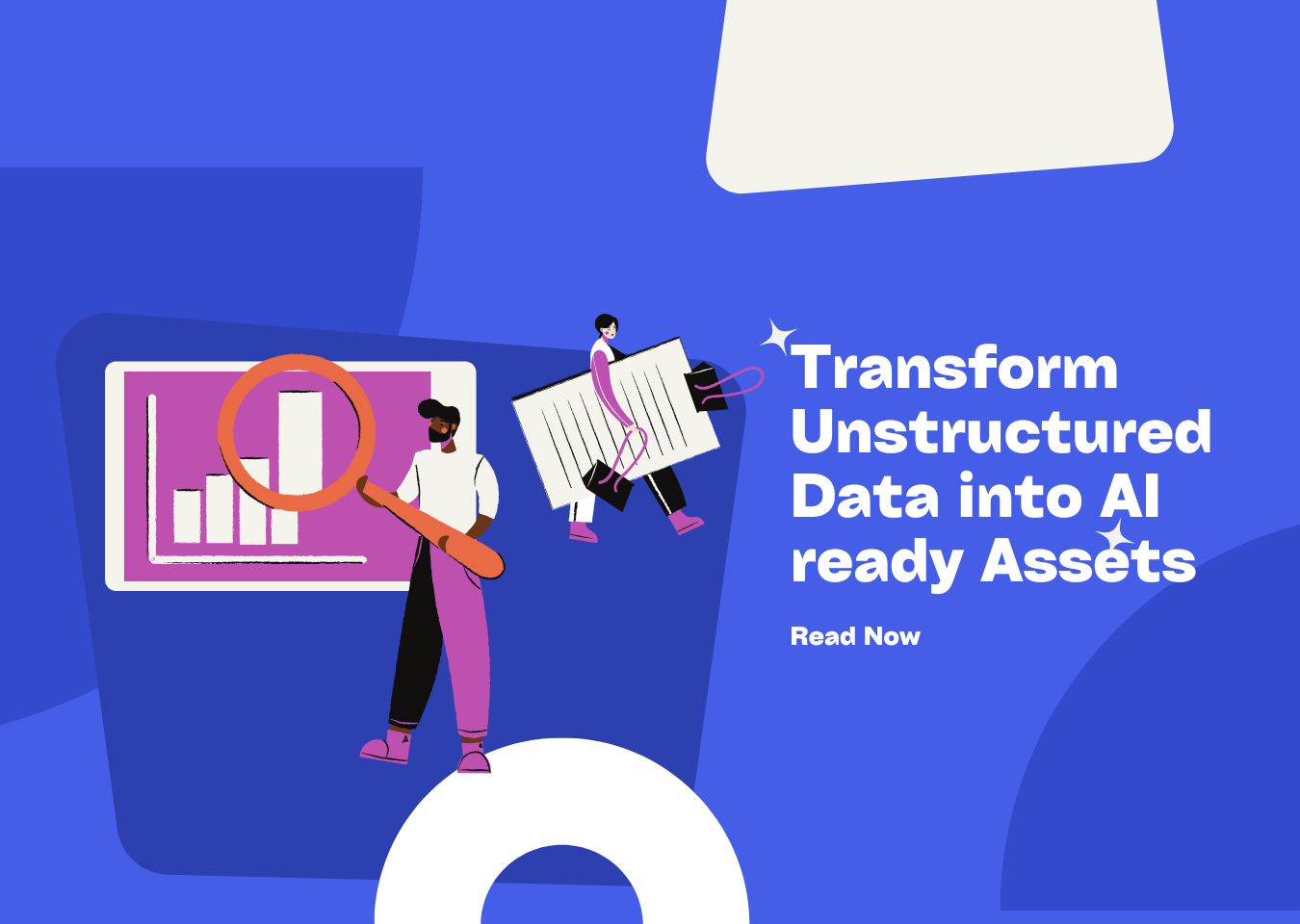
Image Source: unsplash
Unstructured database example dominates the digital landscape, comprising 80% to 90% of all data generated by organizations. This vast amount of information lacks a predefined structure, making it challenging to analyze and manage. You encounter unstructured database example in various forms, such as emails, videos, and social media posts. The rapid growth of this data type demands innovative solutions. Here, AI and machine learning emerge as powerful tools. They help you unlock insights from unstructured database example, transforming it into valuable assets for decision-making and strategic planning.
Understanding Unstructured Database Example
Defining Unstructured Database Example
Unstructured database example refers to information that lacks a predefined format or organization. Unlike structured data, which fits neatly into tables and databases, unstructured database example comes in various forms such as text, images, and videos. You encounter it daily in emails, social media posts, and multimedia files. This type of data is prevalent in today’s digital world, making up a significant portion of the data generated by organizations.
Characteristics and Prevalence
Unstructured database example is characterized by its lack of a specific structure, making it difficult to store and analyze using traditional methods. It often requires advanced tools and techniques for processing. Despite these challenges, unstructured database example is abundant and continues to grow rapidly. Its prevalence is evident in the vast amounts of information shared on social media platforms, through emails, and in multimedia content.
Comparison with Structured and Semi-Structured Data
To understand unstructured database example better, it’s helpful to compare it with structured and semi-structured data. Structured data is organized and easily searchable, typically stored in relational databases. It includes data like names, dates, and numbers. In contrast, semi-structured data falls between structured and unstructured database example. It has a loose schema, often using tags or markers to organize information, making it easier to search than unstructured database example but not as straightforward as structured data.
Challenges of Unstructured Database Example
Unstructured database example presents unique challenges that require innovative solutions. You must address these challenges to effectively manage and utilize this type of data.
Analysis Difficulties
Analyzing unstructured database example can be complex due to its varied formats and lack of organization. Traditional data mining tools struggle to process it, necessitating the use of advanced technologies like AI and machine learning. These tools help you extract valuable insights from unstructured database example, turning it into actionable information.
Storage and Management Issues
Storing and managing unstructured database example poses significant challenges. Unlike structured data, which fits neatly into databases, unstructured database example requires more flexible storage solutions. You might need to use non-relational databases or data lakes to accommodate its diverse formats. Effective management of unstructured database example involves implementing strategies that ensure easy access and retrieval while maintaining data integrity.
Examples of Unstructured Database Example
Image Source: pexels
Human-Generated Data
Human-generated data forms a significant portion of unstructured database example. You encounter it in various everyday activities.
Emails, Text Files, and Social Media
Emails and text files are common examples of unstructured database example. They contain free-form text that lacks a predefined structure. Social media platforms like Twitter and Facebook generate vast amounts of unstructured database example. Posts, comments, and multimedia content such as images and videos fall into this category. This data type is rich in information but requires advanced tools for analysis.
Mobile Data and Media Content
Mobile devices contribute significantly to unstructured database example. You generate data through apps, messages, and multimedia content. Photos, videos, and audio recordings from smartphones are examples of unstructured database example. This data type is diverse and requires innovative solutions for storage and analysis.
Machine-Generated Data
Machine-generated data also contributes to the pool of unstructured database example. It originates from various automated processes and technologies.
Scientific Data and Digital Surveillance
Scientific research generates unstructured database example through experiments and observations. This data includes images, graphs, and reports. Digital surveillance systems produce unstructured database example in the form of video footage and sensor readings. This data type is crucial for security and research purposes.
Satellite Imagery
Satellite imagery is another example of machine-generated unstructured database example. Satellites capture images of the Earth’s surface, providing valuable information for weather forecasting, environmental monitoring, and mapping. Analyzing this data requires specialized tools and techniques.
Analyzing Unstructured Database Example
Role of AI and Machine Learning
AI and machine learning have revolutionized the way you analyze unstructured database example. These technologies provide powerful tools that help you extract meaningful insights from complex datasets.
Emerging Tools and Technologies
Recent advancements in AI have introduced a variety of tools designed to handle unstructured database example. These tools automate tasks, process large volumes of data, and identify hidden patterns with minimal manual intervention. For instance, text mining algorithms powered by AI can sift through vast amounts of text data to uncover trends and relationships. Generative AI models also play a crucial role by creating realistic content and enhancing machine learning processes. These innovations make it easier for you to derive value from unstructured database example, especially in organizations with extensive data reservoirs.
Applications in Big Data Analysis
In the realm of big data, AI and machine learning applications are indispensable. They enable you to analyze unstructured database example efficiently, unlocking insights that drive decision-making. By leveraging these technologies, you can identify emerging trends and potential threats within your data. This capability is vital for organizations aiming to stay competitive in a data-driven world. AI tools not only enhance efficiency but also improve the reliability and accuracy of your analyses.
Structuring Unstructured Database Example
While unstructured database example lacks a predefined format, you can still impose some structure to facilitate analysis and storage.
Internal Structural Elements
To manage unstructured database example effectively, you can identify internal structural elements within the data. These elements might include metadata, tags, or markers that provide context and organization. By recognizing these components, you can create a framework that makes the data more accessible and easier to analyze. This approach helps you transform unstructured database example into a more manageable form without altering its inherent nature.
Use of Non-Relational Databases like MongoDB
Non-relational databases, such as MongoDB, offer flexible solutions for storing unstructured database example. Unlike traditional relational databases, these systems accommodate diverse data formats and structures. MongoDB, for example, allows you to store data in a document-oriented format, making it ideal for handling unstructured database example. This flexibility ensures that you can efficiently manage and retrieve data, aligning with your organization’s needs.
Storage Solutions for Unstructured Database Example
Image Source: pexels
When dealing with unstructured database example, choosing the right storage solution is crucial. You need systems that can handle diverse data formats and provide flexibility for future growth. Let’s explore some effective storage solutions.
NoSQL Databases
NoSQL databases offer a robust solution for managing unstructured database example. They provide flexibility and scalability, making them ideal for handling large volumes of data.
Benefits and Examples
NoSQL databases, like MongoDB, store data in a flexible JSON-like format. This structure allows you to manage vast amounts of unstructured database example efficiently. The horizontal scalability of NoSQL databases ensures that you can expand your storage capacity as needed. Additionally, they offer a rich query language, simplifying data manipulation and retrieval. For instance, InVision, a digital product design platform, uses MongoDB to manage its unstructured database example. With over 5 million users, InVision benefits from MongoDB’s flexible schema and powerful querying options.
MongoDB Atlas for Cloud Storage
MongoDB Atlas provides a cloud-based solution for storing unstructured database example. It offers the same flexibility and scalability as on-premises MongoDB installations but with the added benefits of cloud infrastructure. You can easily manage and scale your data storage without worrying about hardware limitations. MongoDB Atlas also integrates seamlessly with other cloud services, enhancing your ability to analyze and utilize unstructured database example effectively.
Data Lakes and Warehouses
Data lakes and warehouses serve as comprehensive storage solutions for unstructured database example. They allow you to store vast amounts of data in its raw form, providing a foundation for advanced analytics.
Differences and Use Cases
Data lakes and data warehouses differ in their approach to data storage. A data lake stores data in its native format, allowing you to retain all data types, including unstructured database example. This approach is ideal for organizations that need to perform complex analyses on diverse datasets. In contrast, a data warehouse organizes data into structured formats, making it easier to query and analyze. While data warehouses are more suited for structured data, they can still accommodate unstructured database example with some preprocessing.
Integration with Existing Systems
Integrating data lakes and warehouses with your existing systems enhances your ability to manage unstructured database example. You can leverage these storage solutions to consolidate data from various sources, creating a unified data repository. This integration streamlines data access and analysis, enabling you to derive valuable insights from your unstructured database example. By aligning your storage solutions with your organizational needs, you ensure that your data management strategy supports your business objectives.
Effective Strategies for Managing Unstructured Database Example
Effectively managing unstructured database example requires strategic implementation of AI and machine learning, along with selecting the right storage solutions. These strategies help you harness the full potential of your data, turning it into a valuable resource for your organization.
Implementing AI and Machine Learning
AI and machine learning play a crucial role in managing unstructured database example. They offer advanced capabilities that simplify data processing and analysis.
Best Practices and Considerations
When implementing AI and machine learning, consider the following best practices:
- Data Quality: Ensure your data is clean and accurate. High-quality data improves the reliability of AI models.
- Scalability: Choose scalable AI solutions that can grow with your data needs. This ensures long-term efficiency.
- Integration: Seamlessly integrate AI tools with your existing systems. This enhances data flow and usability.
Data Governance Expert: ”Best practices for unstructured data management include maintaining data quality and ensuring scalability in AI solutions.”
Real-world Applications and Case Studies
AI and machine learning have transformed various industries by providing innovative solutions for unstructured database example challenges. For example:
- Healthcare: AI analyzes medical images to detect diseases early, improving patient outcomes.
- Retail: Machine learning algorithms personalize customer experiences by analyzing purchase history and preferences.
These applications demonstrate the power of AI and machine learning in extracting valuable insights from unstructured database example.
Choosing the Right Storage Solution
Selecting the appropriate storage solution is vital for managing unstructured database example effectively. It ensures data accessibility and security.
Factors to Consider
When choosing a storage solution, consider these factors:
- Data Volume: Assess the amount of data you need to store. This helps determine the required storage capacity.
- Access Speed: Evaluate how quickly you need to access your data. Faster access speeds enhance productivity.
- Security: Ensure your storage solution provides robust security features to protect sensitive data.
Aligning with Organizational Needs
Align your storage solution with your organization’s specific needs. Consider:
- Budget: Choose a cost-effective solution that fits your financial constraints.
- Compliance: Ensure the solution complies with industry regulations and standards.
- Future Growth: Select a solution that can accommodate future data growth, ensuring long-term viability.
By implementing AI and machine learning and choosing the right storage solutions, you can effectively manage unstructured database example. These strategies empower you to unlock the full potential of your data, driving innovation and success in your organization.
You have explored effective strategies for managing unstructured database example. Key points include understanding its characteristics, leveraging AI and machine learning, and selecting appropriate storage solutions. To manage unstructured database example effectively, consider these actionable insights:
- Utilize Advanced Tools: Implement AI technologies like NLP and computer vision to extract meaningful insights.
- Choose Flexible Storage: Opt for NoSQL databases or data lakes to accommodate diverse data formats.
- Adopt Strategic Approaches: Align your data management strategies with organizational goals.
Adopting suitable tools and strategies is crucial. They empower you to transform unstructured database example into valuable assets, driving innovation and informed decision-making.
📖See Also
- Demystifying-Unstructured-Data-Analysis-A-Complete-Guide
- Cracking-Document-Parsing-Technologies-and-Datasets-for-Structured-Information-Extraction
- Comparison-of-API-Services-Graphlit-LlamaParse-UndatasIO-etc-for-PDF-Extraction-to-Markdown
- Comparing-Top-3-Python-PDF-Parsing-Libraries-A-Comprehensive-Guide
- Assessment-Unveiled-The-True-Capabilities-of-Fireworks-AI
- Assessment-of-Microsofts-Markitdown-series2-Parse-PDF-files
- Assessment-of-MicrosoftsMarkitdown-series1-Parse-PDF-Tables-from-simple-to-complex
- AI-Document-Parsing-and-Vectorization-Technologies-Lead-the-RAG-Revolution
Subscribe to Our Newsletter
Get the latest updates and exclusive content delivered straight to your inbox